Table Of Content
- Selecting and Improving Quasi-Experimental Designs in Effectiveness and Implementation Research
- Knowledge and skills for financial decision making-Low-Favorable impacts
- Employ a model or framework that covers both internal and external validity
- The Use and Interpretation of Quasi-Experimental Studies in Medical Informatics
- Explanatory Research – Types, Methods, Guide
- Quasi-experimental Designs without Control Groups
- QUASI-EXPERIMENTAL DESIGNS FOR PROSPECTIVE EVALUTION OF INTERVENTIONS
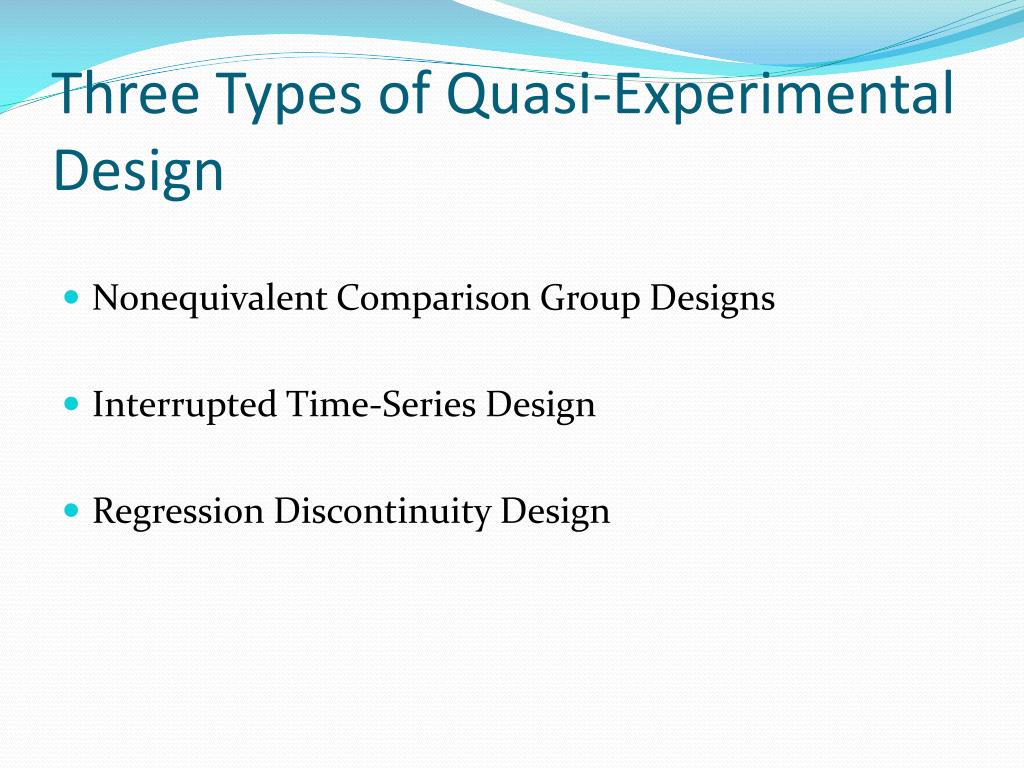
In addition to including a control group, several analysis phase strategies can be employed to strengthen causal inference including adjustment for time varying confounders and accounting for auto correlation. The directionality problem is avoided in quasi-experimental research since the regression analysis is altered before the multiple regression is assessed. However, because individuals are not randomized at random, there are likely to be additional disparities across conditions in quasi-experimental research. Researchers assessed the program's effectiveness by assigning the selected subjects to a randomly assigned treatment group, while those that didn't win the lottery were considered the control group. This study didn't use specific aspects to determine which start-up companies should participate. Therefore, the results may seem straightforward, but several aspects may determine the growth of a specific company, apart from the variables used by the researchers.
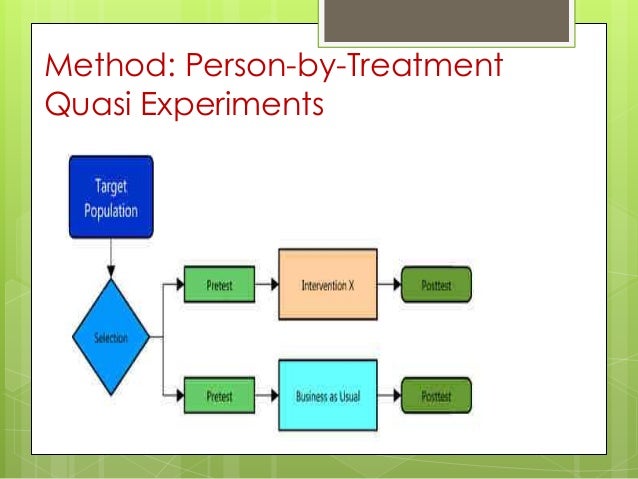
Selecting and Improving Quasi-Experimental Designs in Effectiveness and Implementation Research
Here, X is the intervention and O is the outcome variable (this notation is continued throughout the article). In this study design, an intervention (X) is implemented and a posttest observation (O1) is taken. For example, X could be the introduction of a pharmacy order-entry intervention and O1 could be the pharmacy costs following the intervention. This design is the weakest of the quasi-experimental designs that are discussed in this article. Without any pretest observations or a control group, there are multiple threats to internal validity.
Knowledge and skills for financial decision making-Low-Favorable impacts
A social network intervention to improve connectivity and burnout among psychiatry residents in an academic institution ... - BMC Medical Education
A social network intervention to improve connectivity and burnout among psychiatry residents in an academic institution ....
Posted: Fri, 13 May 2022 07:00:00 GMT [source]
Commonly used in medical informatics (a field that uses digital information to ensure better patient care), researchers generally use this design to evaluate the effectiveness of a treatment – perhaps a type of antibiotic or psychotherapy, or an educational or policy intervention. With random assignment, study participants have the same chance of being assigned to the intervention group or the comparison group. As a result, differences between groups on both observed and unobserved characteristics would be due to chance, rather than to a systematic factor related to treatment (e.g., illness severity). The study used a nonexperimental design to assess the impact of a workplace financial education program. Two companies offered a financial education program to all employees for a one-time reimbursed fee of $150. The program group consisted of 46 employees, 10 from Company A and 36 from Company B. Program participants attended classes once a week over a 10-week period.
Employ a model or framework that covers both internal and external validity
Even though quasi-experimental design has been used for some time, relatively little is known about it. This method involves summarizing the data collected during a study using measures such as mean, median, mode, range, and standard deviation. Descriptive statistics can help researchers identify trends or patterns in the data, and can also be useful for identifying outliers or anomalies. Discover the concept of quasi-experiment, its various types, real-world examples, and how QuestionPro aids in conducting these studies.
The Use and Interpretation of Quasi-Experimental Studies in Medical Informatics
Quasi-experimental research designs play a vital role in scientific inquiry by allowing researchers to investigate cause-and-effect relationships in real-world settings. These designs offer practical and ethical alternatives to true experiments, making them valuable tools in various fields of study. With their versatility and applicability, quasi-experimental designs continue to contribute to our understanding of complex phenomena. Traditionally, experimental researchers have used convenience sampling to select study participants. However, as research methods have become more rigorous, and the problems with generalizing from a convenience sample to the larger population have become more apparent, experimental researchers are increasingly turning to random sampling. In experimental policy research studies, participants are often randomly selected from program administrative databases and randomly assigned to the control or treatment groups.
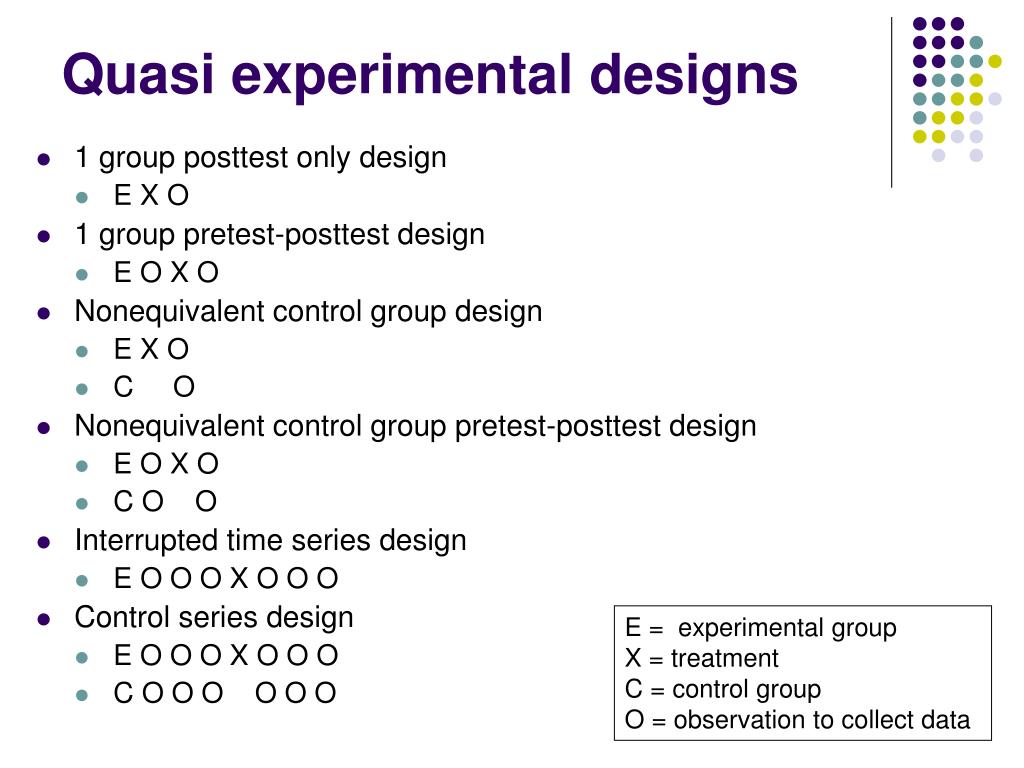
Explanatory Research – Types, Methods, Guide
An interrupted time-series design is one in which a string of consecutive observations equally spaced in time is interrupted by the imposition of a treatment or intervention. The advantage of this design is that with multiple measurements both pre- and postintervention, it is easier to address and control for confounding and regression to the mean. In the example of a pharmacy order-entry system, O1 through O5 could represent monthly pharmacy costs preintervention and O6 through O10 monthly pharmacy costs post the introduction of the pharmacy order-entry system. Interrupted time-series designs also can be further strengthened by incorporating many of the design features previously mentioned in other categories (such as removal of the treatment, inclusion of a nondependent outcome variable, or the addition of a control group).
How Survey Software is Revolutionizing Data Collection in Market Research
In Cambodia, the evaluators used DDD analysis to evaluate the Cambodia Education Sector Support Project, overcoming the observed lack of common trends in preprogram outcomes between beneficiaries and nonbeneficiaries [26]. Specific objectives were (1) to include a question to capture information about clustering; and (2) to extend the checklist to include study designs often used by health systems researchers and econometricians in a way that deals with the design/analysis challenge. We intended that the revised checklist should be able to resolve the differences in opinion about the extent to causality can be inferred from nonrandomized studies with different design features, improving communication between different health research communities. We did not intend that the checklist should be used as a tool to assess risk of bias, which can vary across studies with the same design features.
Quasi-experimental Designs without Control Groups
Quasi-experimental designs can also help inform policy and practice by providing valuable insights into the causal relationships between variables. The use of a comparison group helps prevent certain threats to validity including the ability to statistically adjust for confounding variables. Because in this study design, the two groups may not be equivalent (assignment to the groups is not by randomization), confounding may exist. For example, suppose that a pharmacy order-entry intervention was instituted in the medical intensive care unit (MICU) and not the surgical intensive care unit (SICU). O1 would be pharmacy costs in the MICU after the intervention and O2 would be pharmacy costs in the SICU after the intervention.
(PDF) Examining the effectiveness of Technology use by Educators to improve students' Test Scores: A Quasi ... - ResearchGate
(PDF) Examining the effectiveness of Technology use by Educators to improve students' Test Scores: A Quasi ....
Posted: Sat, 06 Jun 2020 04:44:50 GMT [source]
This design can be useful for determining whether the intervention or event had an effect, but it does not allow for control over other factors that may have influenced the outcomes. The quality of causal evidence presented in this study is low because the authors did not ensure that the groups being compared were similar before the intervention. This means we are not confident that the estimated effects are attributable to financial education in the workplace; other factors are likely to have contributed.
Detailed reviews have been published of variations on the basic ITS design that can be used to enhance causal inference. In particular, the addition of a control group can be particularly useful for assessing for the presence of seasonal trends and other potential time-varying confounders (52). Zombre et al (52) maintained a large number of control number of sites during the extended study period and were able to look at variations in seasonal trends as well as clinic-level characteristics, such as workforce density and sustainability.
In this design, all subjects receive the new PDA-based order entry system and the old computer terminal-based order entry system. The counterbalanced design is a within-participants design, where the order of the intervention is varied (e.g., one group is given software A followed by software B and another group is given software B followed by software A). The counterbalanced design is typically used when the available sample size is small, thus preventing the use of randomization. This design also allows investigators to study the potential effect of ordering of the informatics intervention. In the four-year period of JAMIA publications that the authors reviewed, 25 quasi-experimental studies among 22 articles were published.
Public health practice involves implementation or adaptation of evidence-based interventions into new settings in order to improve health for individuals and populations. Such interventions typically include on one or more of the “7 Ps” (programs, practices, principles, procedures, products, pills, and policies) (9). Increasingly, both public health and clinical research have sought to generate practice-based evidence on a wide range of interventions, which in turn has led to a greater focus on intervention research designs that can be applied in real-world settings (2, 8, 9, 20, 25, 26, 10, 2). Quasi-experimental research designs are a type of research design that is similar to experimental designs but doesn’t give full control over the independent variable(s) like true experimental designs do. Much like an actual experiment, quasi-experimental research tries to demonstrate a cause-and-effect link between a dependent and an independent variable.
The checklist shows that credible quasi-experimental studies gain credibility from using high-quality longitudinal/panel data; such data characterizing health care are rare, leading to evaluations that “make do” with the data that are available in existing information systems. The advantage of this design is that it demonstrates reproducibility of the association between the intervention and the outcome. For example, the association is more likely to be causal if one demonstrates that a pharmacy order-entry system results in decreased pharmacy costs when it is first introduced and again when it is reintroduced following an interruption of the intervention. As for design A5, the assumption must be made that the effect of the intervention is transient, which is most often applicable to medical informatics interventions.
In the study by Zombré et al (52) on health care access in Burkina Faso, the authors examined clinic density characteristics to determine its impact on sustainability. While the basic ITS design has important strengths, the key threat to internal validity is the possibility that factors other than the intervention are affecting the observed changes in outcome level or trend. Changes over time in factors such as the quality of care, data collection and recording, and population characteristics may not be fully accounted for by the pre-intervention trend. Similarly, the pre-intervention time period, particularly when short, may not capture seasonal changes in an outcome. But at the same time there is a control group that is given a pretest, does not receive the treatment, and then is given a posttest. The question, then, is not simply whether participants who receive the treatment improve but whether they improve more than participants who do not receive the treatment.
Quasi-experiments are commonly used in social sciences, public health, education, and policy analysis, especially when it is not practical or reasonable to randomize study participants to the treatment condition. The purpose of quasi-experimental design is to investigate the causal relationship between two or more variables when it is not feasible or ethical to conduct a randomized controlled trial (RCT). Quasi-experimental designs attempt to emulate the randomized control trial by mimicking the control group and the intervention group as much as possible. The treatment is that the instructor begins publicly taking attendance each day so that students know that the instructor is aware of who is present and who is absent. There is a consistently high number of absences before the treatment, and there is an immediate and sustained drop in absences after the treatment. This figure also illustrates an advantage of the interrupted time-series design over a simpler pretest-posttest design.
In one classic example, the treatment was the reduction of the work shifts in a factory from 10 hours to 8 hours (Cook & Campbell, 1979). As we mentioned before, quasi-experimental research entails manipulating an independent variable by randomly assigning people to conditions or sequences of conditions. Non-equivalent group designs, pretest-posttest designs, and regression discontinuity designs are only a few of the essential types. In a quasi-experimental design, the researcher uses an existing group of participants that is not randomly assigned to the experimental and control groups. Instead, the groups are selected based on pre-existing characteristics or conditions, such as age, gender, or the presence of a certain medical condition. Quasi-experimental methods are used increasingly to evaluate programs in health systems research.